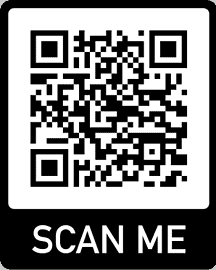
Which time frame performing the best result after comparing the sharpe vs sortino ratio?
Test EMA Strategy 1:
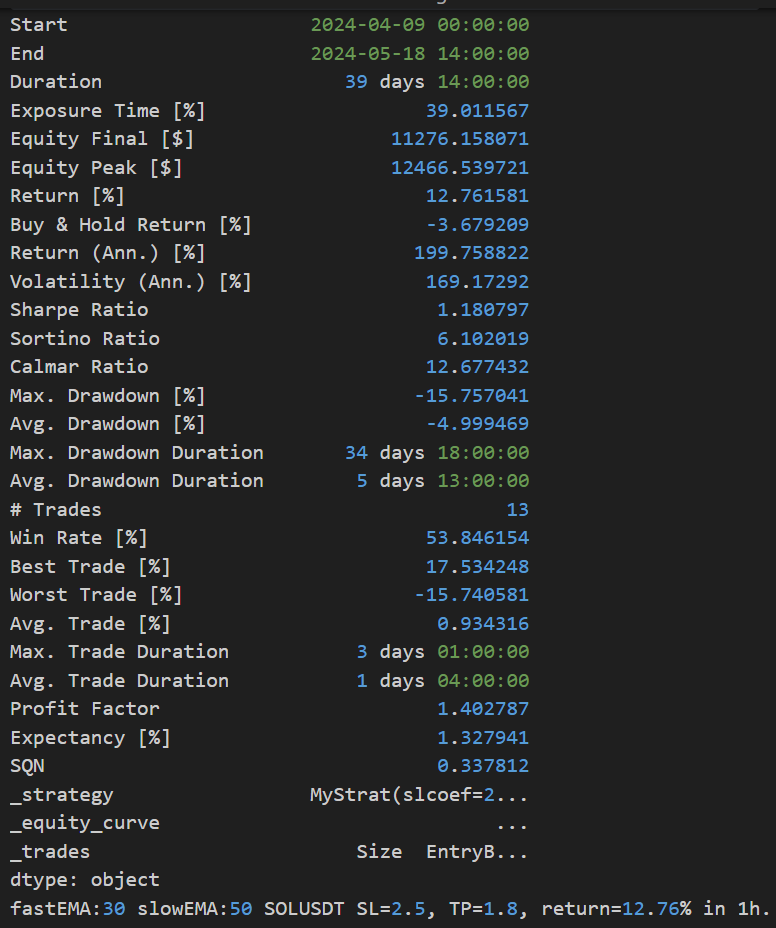
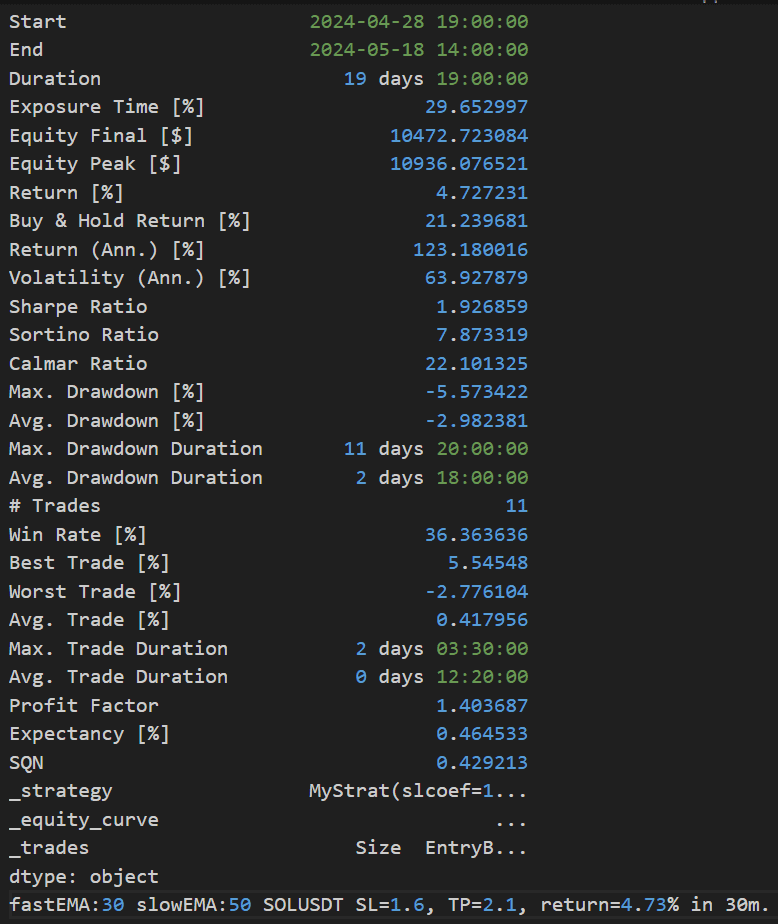
Test SMA Strategy 2:
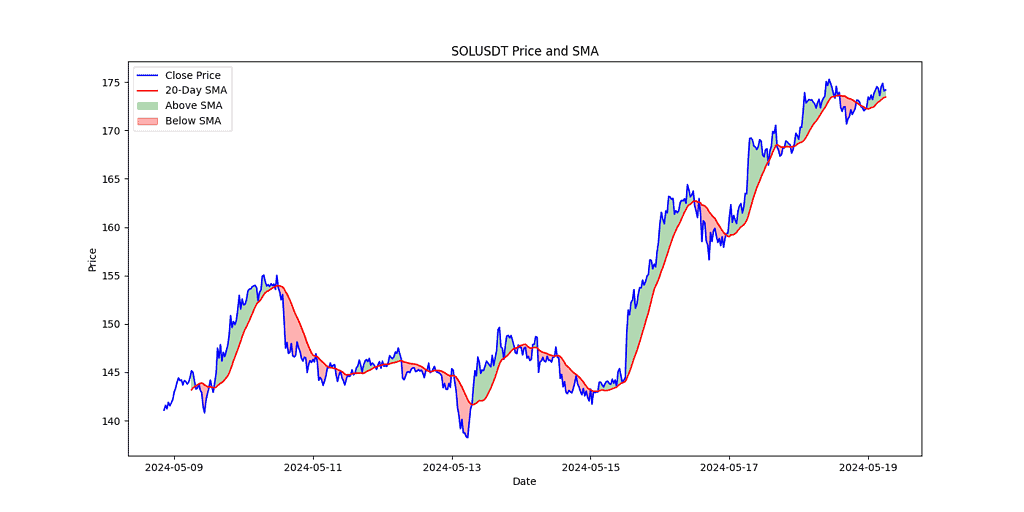
DGM Class Start Date and Time now is 2024-05-19 14:18:50.497258
START: 30m SOLUSDT OHLC-data from 2020-11-11 00:00:00 until 2024-05-19
DGM Class Start Date and Time now is 2024-05-19 14:22:57.747713
START: 30m SOLUSDT OHLC-data from 2020-11-11 00:00:00 until 2024-05-19
Open Time Open High Low Close
0 2020-11-10 16:00:00 2.1840 2.2528 2.1839 2.2484
1 2020-11-10 16:30:00 2.2484 2.2485 2.2304 2.2409
2 2020-11-10 17:00:00 2.2395 2.2995 2.2255 2.2790
3 2020-11-10 17:30:00 2.2790 2.2869 2.2438 2.2554
4 2020-11-10 18:00:00 2.2518 2.2554 2.2111 2.2111
.. … … … … …
659 2024-05-19 04:00:00 174.3200 174.4100 173.3100 173.5900
660 2024-05-19 04:30:00 173.5900 174.5000 173.3500 174.5000
661 2024-05-19 05:00:00 174.5000 175.5000 174.3600 174.8500
662 2024-05-19 05:30:00 174.8600 174.9400 173.8600 174.0700
663 2024-05-19 06:00:00 174.0700 174.3400 173.9200 174.1400
[61664 rows x 5 columns]
END: v3/klines 30m SOLUSDT DB Processed.
START DB Processing: >>> Data from GetDataRequested table.
Mean Price: 62.08541965814739
Standard Deviation: 58.721715230338866
v3/klines Upper Percentage Threshold: 179.52885011882512
v3/klines Lower Percentage Threshold: -55.358010802530345
Open Time High Low Close
0 2020-11-10 16:00:00 2.2528 2.1839 2.2484
1 2020-11-10 16:30:00 2.2485 2.2304 2.2409
2 2020-11-10 17:00:00 2.2995 2.2255 2.2790
3 2020-11-10 17:30:00 2.2869 2.2438 2.2554
4 2020-11-10 18:00:00 2.2554 2.2111 2.2111
GetDataProcess Trade Message = None
GetDataProcess Trade Price (Qty: 0.05) = 0
GetDataProcess Data processed and saved to QuantTrending table.
END: v3/klines 30m SOLUSDT GetDataProcess Completed.
START DB Processing: >>> Data from QuantTrending table.
BackTestSharpRatioMDD: >>> Processed the file QuantTrending.csv.
Warning: NaN values detected in ‘Previous_Close’. Attempting to recalculate ‘Daily_PnL’.
Warning: NaN or Infinite values detected in ‘Daily_PnL’ after recomputation. Cleaning required.
Info: Previous_Close Close Daily_PnL Cumm_PnL
count 61663.000000 61663.000000 61663.000000 61663.000000
mean 62.083602 62.086390 0.001621 63.921336
std 58.720934 58.722173 0.007704 26.552746
min 1.101800 1.101800 -0.178662 0.000000
25% 20.810000 20.810000 0.000000 49.072912
50% 33.951000 33.960000 0.000000 72.006780
75% 97.620000 97.620000 0.000000 85.162826
max 258.440000 258.440000 0.200871 99.982746
*> DGM Sharp Ratio = 4.02
**> DGM Maximum Drawdown: 17.87%
***> DGM Peak Profit: 9998.27%
****> DGM Drawdown from Peak: 0.00%
*****>> Created SOLUSDT-QuantSharpRatioMDD-30m.csv for Equity Curve.
Test LWMA Strategy 3:
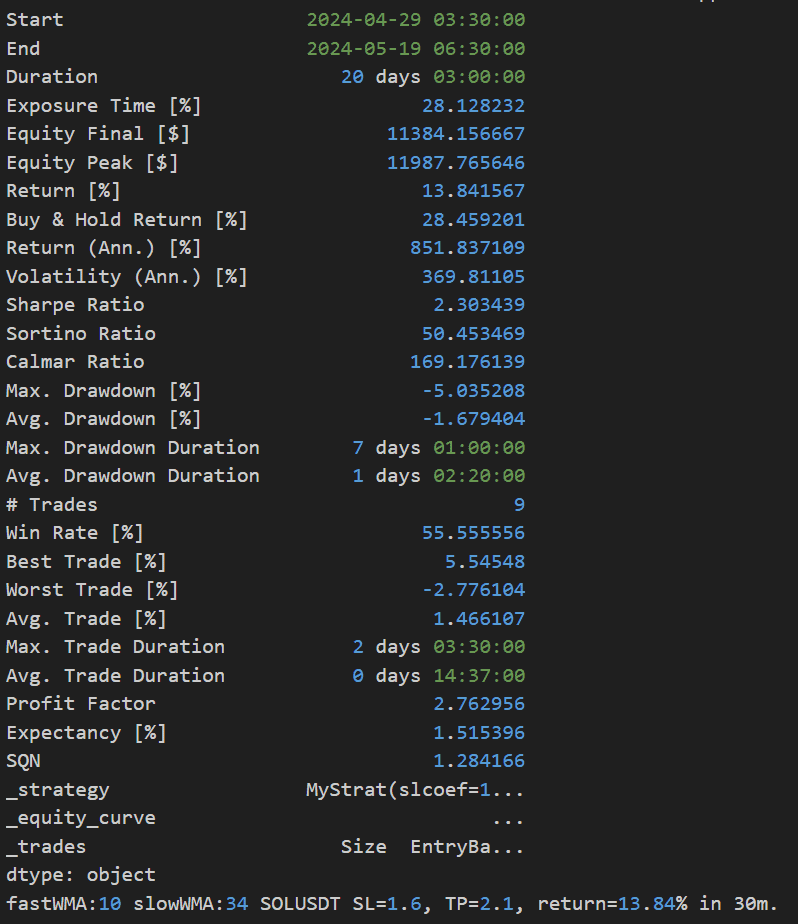
In summary:
fastEMA:30 slowEMA:50 SOLUSDT SL=2.5, TP=1.8, return=12.76% in 1h.
fastEMA:30 slowEMA:50 SOLUSDT SL=1.6, TP=2.1, return=4.73% in 30m.
fastEMA:30 slowEMA:50 SOLUSDT SL=1.6, TP=2.1, return=4.30% in 30m.
fastWMA:10 slowWMA:34 SOLUSDT SL=1.6, TP=2.1, return=13.84% in 30m.
In 1993, Buffett spoke to Columbia University’s Business School graduates. Asked about his method for evaluating risk, he said, “Risk comes from not knowing what you’re doing.” This quote reflects Buffett’s investment philosophy, highlighting the crucial role of knowledge and understanding in reducing risk.
“The biggest risk is not taking any risk… In a world that changing really quickly, the only strategy that is guaranteed to fail is not taking risks.” Mark Zuckerberg
- Free VPN by Cloudflare (DNS)
- 3 SOP In Trading
- 2024 Building A Strong Heart With Quant Trading
- 2024 Building A Strong Heart With AI
- 2024 December Achievements
- Happy Achievements
Tips:
Despite of the crypto dump recently on all the alt coins after SEC announcement to sue Binance and Coinbase. Guess what? My Ai Trading Strategies are making shit ton of USDT from the crazy markets. Well there is a secret and cannot tell you unless…Anyway, I have given you the formula to copy and it is up to you to trade manually with stress and sleepless nights or ride on the trend of Ai trading today ⬇️⬇️⬇️
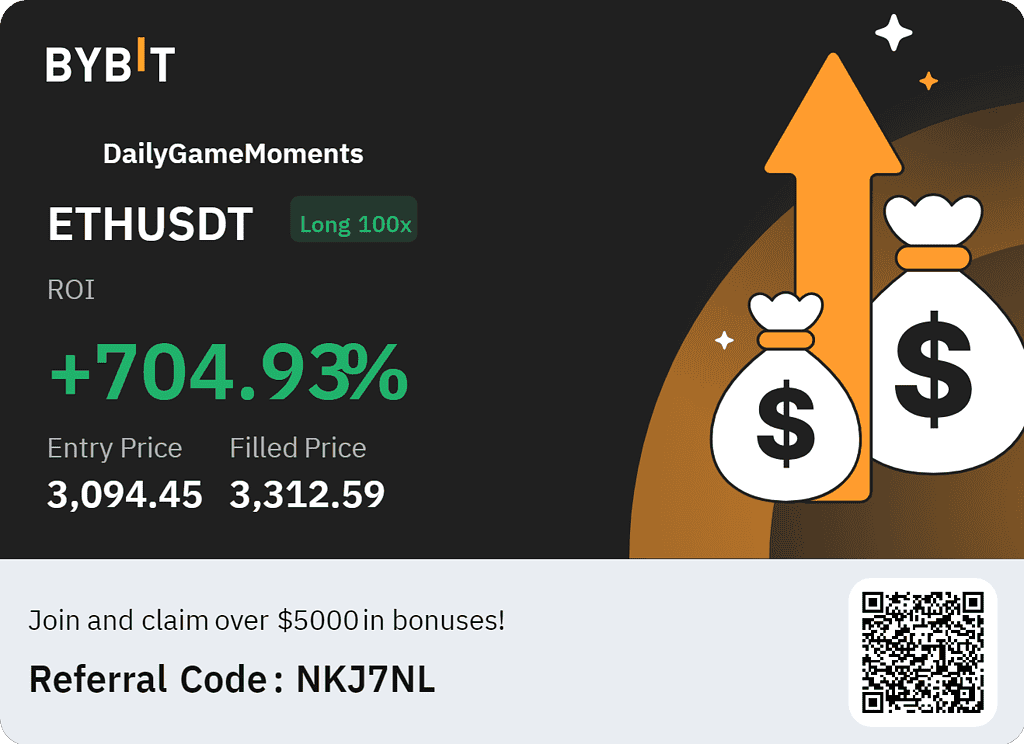
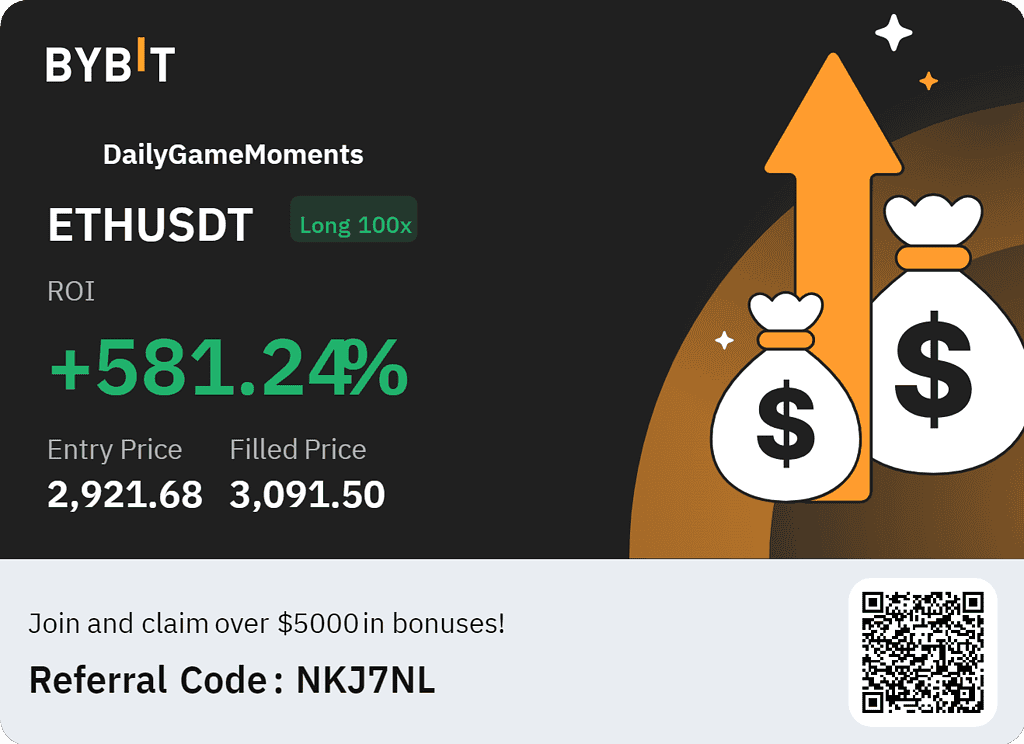
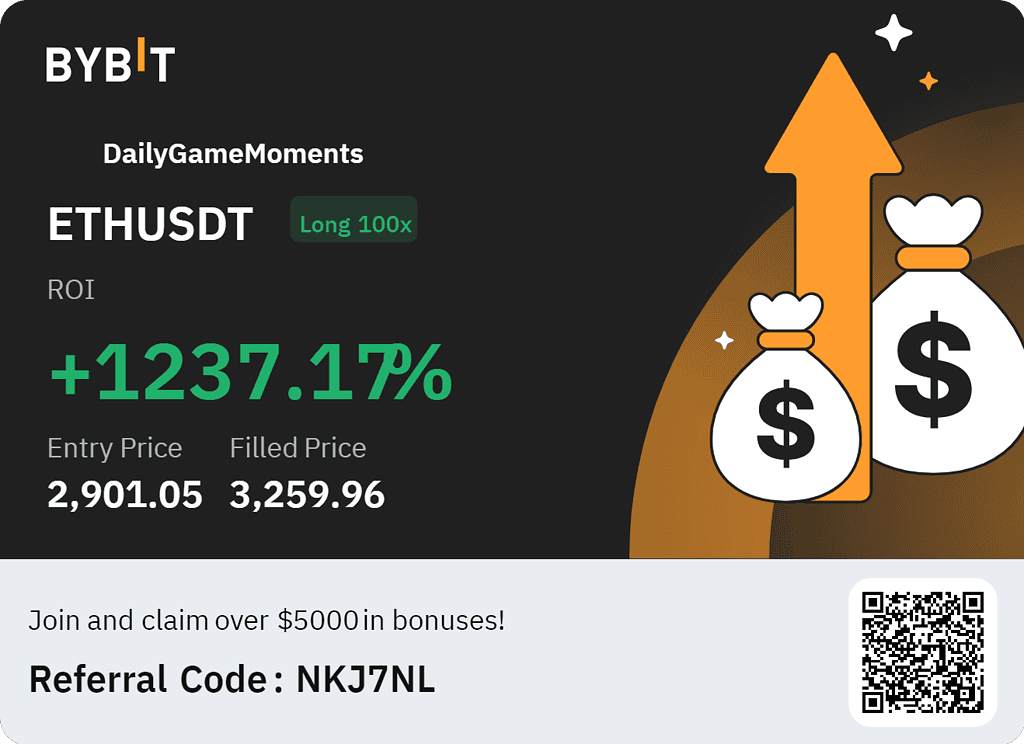
AI Sleeping Income With DGM System
The SECRET is to marry between Ai trading strategies and an income generated exchange platform
- Ai trading strategies
- An income generated exchange platform